UrbanPipe Dataset
UrbanPipe: A fine-grained and multi-labeled Video Dataset collected from various Quick-View (QV) Inspection devices in the real-world urban pipe systems.
 
Xuan Zhang  
 
Yi Liu  
 
Ying Li  
 
Guixin Liang  
 
Fei Xie  
 
Wei Yao  
 
Yi Dai  
 
Yali Wang  
 
Yu Qiao  
MMLAB @ Shenzhen Institute of Advanced Technology
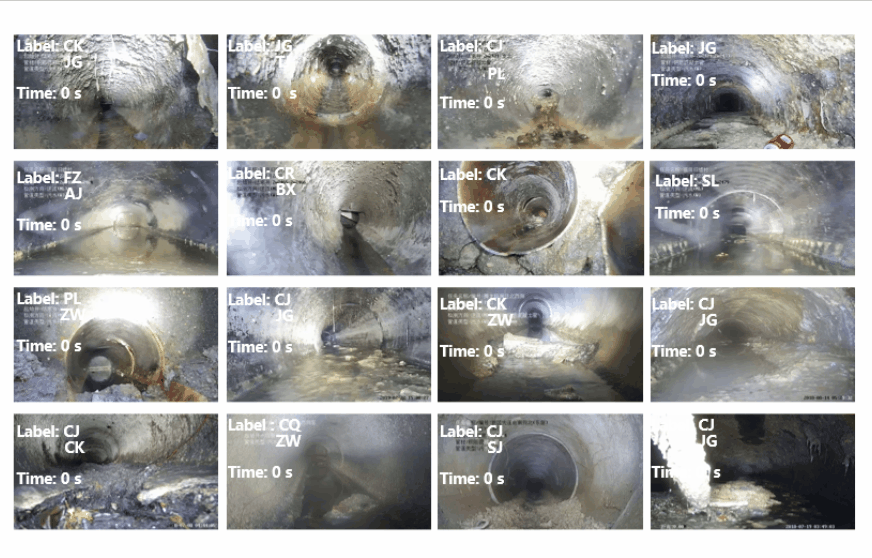
Abstract
Video anomaly analysis is important for industrial applications in the real world. In particular, the urban pipe system is one of the most important infrastructures in a city. In order to ensure its normal operation, we need to inspect pipe defects smartly. For video anomaly recognition, we focus on fine-grained and multi-labeled defect recognition in the real-world urban systems. We have collected a new dataset, termed as UrbanPipe. UrbanPipe is collected from various Quick-View (QV) Inspection devices in the real-world urban pipe systems. It consists of 9.6k short videos with 16 anomaly classes, which are collected from real-world urban pipes and annotated by professional engineers. The total duration of all videos exceeds 55 hours.
Data Collection & Annotation
The UrbanPipe dataset consists of 1 normal class and 16 defect classes and 9.6k videos, which are collected from real-world urban pipes and annotated by professional engineers. The total duration of all videos exceeds 55 hours. Because the pipe situation is complex, multiple defects often appear at the same time, so each video is annotated by multiple labels. To obtain accurate annotations of defect instances, professional engineers are asked to check all the videos multiple rounds with cross validation.
Dataset Statitics
The UrbanPipe video duration ranges from 0.7 seconds to 177.4 seconds. Each video is annotated by 1 to 5 categories. On average, each video has the duration of 20.7 seconds and 1.4 labels. The 9.6k videos are divided into train set and test set according to the ratio of 2:1. As shown in Figure 2, the data exhibits the natural long-tailed distribution.
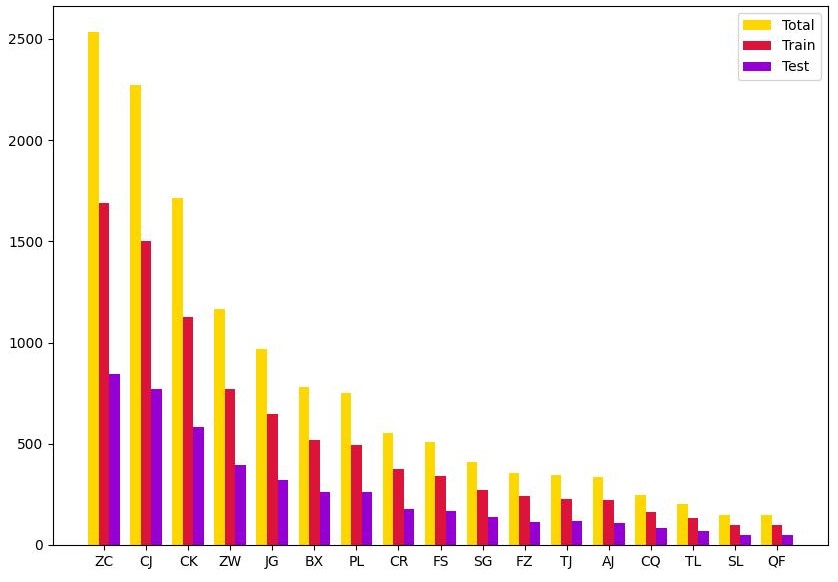
Figure 1. Data Distribution of UrbanPipe
Download
Please refer to the competition page for more information.