FineAction Dataset
FineAction: A Fine-Grained Video Dataset for Temporal
Action Localization
[paper]
  Yi Liu     Limin Wang     Xiao Ma     Yali Wang     Yu Qiao  
MMLAB @ Shenzhen Institute of Advanced Technology
MCG Group @ Nanjing University
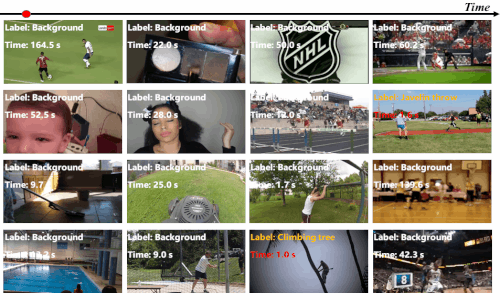
Abstract
Temporal action localization (TAL) is an important and challenging problem in video understanding. However, most existing TAL benchmarks are built upon the coarse granularity of action classes, which exhibits two major limitations in this task. First, coarse-level actions can make the localization models overfit in high-level context information, and ignore the atomic action details in the video. Second, the coarse action classes often lead to the ambiguous annotations of temporal boundaries, which are inappropriate for temporal action localization. To tackle these problems, we develop a novel large-scale and fine-grained video dataset, coined as FineAction, for temporal action localization. In total, FineAction contains 103K temporal instances of 106 action categories, annotated in 17K untrimmed videos. FineAction introduces new opportunities and challenges for temporal action localization, thanks to its distinct characteristics of fine action classes with rich diversity, dense annotations of multiple instances, and co-occurring actions of different classes. To benchmark FineAction, we systematically investigate the performance of several popular temporal localization methods on it, and deeply analyze the influence of short-duration and fine-grained instances in temporal action localization. We believe that FineAction can advance research of temporal action localization and beyond.
FineAction Taxonomy
We generate 106 action classes within a new taxonomy of three-level granularity. This taxonomy consists of 4 top-level categories (Household Activities, Personal Care, Socializing, Relaxing and Sports, Exercise), 14 middle-level categories, and 106 bottom-level categories.
- Complete organizational taxonomy behind FineAction.
Dataset Statitics
Our FineAction is a large-scale dataset that is suitable for training deep learning models, which contains 103,324 instances for a total of 705 video hours. As a consequence, the number of instances in FineAction is as great as 6.17 per video and 975 per category.
- Comparison with Related Benchmarks. Our FineAction is unique due to its fine-grained
action classes, multi-label and dense annotations, relatively large-scale capacity, and
rich action diversity.
- Number of instances per category. We plot the instance distribution of all the
bottom-level categories in each top-level category. All the plots exhibit the natural
long-tailed distribution.
Experiment Results
- Comparison of the state-of-the-art methods on the validation set of FineAction. Left:
evaluation on action proposal generation, in terms of AR@AN. Right : evaluation on
action detection, in terms of mAP at IoU thresholds from 0.5 to 0.95.
- Error analysis on FineAction. (a) Left : the error distribution over the number of
predictions per video. G means the number of Ground-Truth instances. Right: the impact
of error types, measured by the improvement gained from resolving a particular type of
error. (b) Visualization of typical failure cases on FineAction.
Download
Please refer to the competition page for more information.